PhD Student Spotlight: Ziyuan Wang
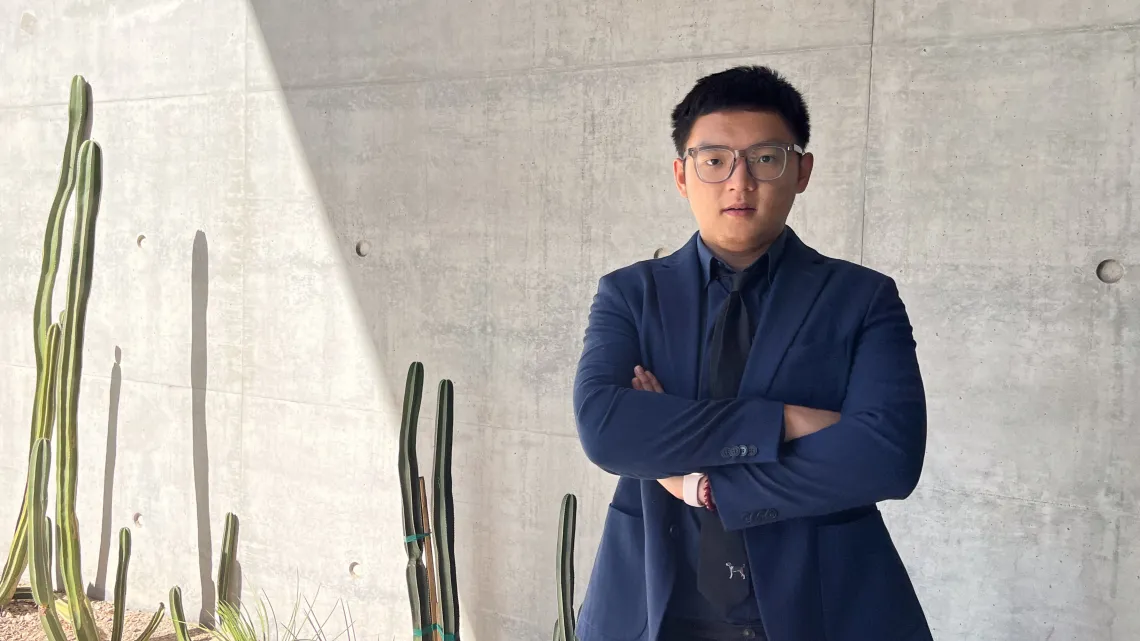
Ziyuan Wang received his Bachelor's Degree from Sichuan University in 2022 and is currently a third-year PhD student. His research focuses on nanopore sequencing under the mentorship of Hongxu Ding, PhD, where he developed IL-AD software for RNA modification detection. In his spare time, he enjoys playing soccer and tennis.
Q & A
How do you think technological advancements will affect the future of pharmacy practice?
- Currently, I think Nanopore Sequencing will make a difference, because this device is a portable, cheap and fast detection method. It will make a difference in precision medicine and drug development. Plus, AI will also change the pharmacy practice as now chatGPT has changed the world.
What’s your favorite spot on campus to relax or study?
- Recreation Center. Doing sports definitely helps me relax and become more energetic.
What’s the most memorable event or tradition at the College of Pharmacy?
- Homecoming Event! A lot of alumni will come back and give us advice.
What’s the best piece of advice you’ve received from a professor or mentor?
- Dr. Qin Chen (Holsclaw Endowed Pharmacogenomics Professor, Pharmacogenomics Director) told me to pay special attention to detailed things. Never be sloppy.
What’s one study habit or routine that has been essential to your success in pharmacy school?
- Before I begin to work, I will use online notes to list a plan. When some insights or important knowledge come, I will instantly describe them in that note.
Published Research
Recently, Wang had a paper published in Nature Communications. Adapting nanopore sequencing basecalling models for modification detection via incremental learning and anomaly detection was co-authored by University of Arizona collaborators Ziyang Liu, a fourth-year PhD student in GIDP statistics and data science program; Ning Hao, PhD, an associate professor of Mathematics; Hao Helen Zhang, PhD, professor and chair of statistics and data science GIDP; Xiaoxiao Sun, an associate professor at the Mel and Enid Zuckerman College of Public Health; and from Columbia University Medical Center, Yinshan Fang, PhD, and Jianwen Que, PhD.
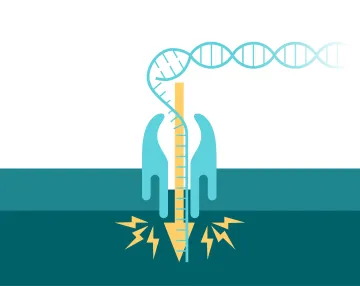
The team developed a new method to improve how Nanopore sequencing detects modifications in RNA and DNA. This tool known as IL-AD enables more accurate detection of nucleotide modifications, particularly in regions with numerous modifications, thereby advancing molecular and genetic research. The research was led by Hongxu Ding, PhD, an Assistant Professor at the R. Ken Coit College of Pharmacy.
Nanopore Sequencing is a cutting-edge technology that enables real-time, single-molecule sequencing by passing DNA or RNA strands through a Nanopore. As the nucleic acids move through the pore, changes in electrical current are detected. Then the signal will be translated into nucleotide sequences by deep learning algorithms known as basecallers. This technology is particularly valued for long-read sequencing and modification detection in nucleic acids, but challenges remain, especially when it comes to accurately identifying sequences with a high density of modifications.
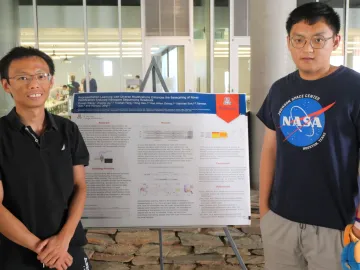
Dr. Hongxu Ding (right) stands with Ziyuan Wang (right).
To address these challenges, Ding, and his team employed a novel approach by first implementing incremental learning to tackle the issues posed by modification-disturbed basecalling. This technique significantly enhances the accuracy of sequencing for modification-rich sequences, which are often of high biological interest. Once the sequence backbones were resolved, the researchers utilized anomaly detection to evaluate the modification status of individual nucleotides.
This innovative pipeline offers a powerful solution for the single-molecule, single-nucleotide detection of modifications. The team rigorously benchmarked their approach using control oligos and applied it to various complex biological systems. This included the basecalling of densely-modified yeast tRNAs and E. coli genomic DNA, cross-species detection of N6-methyladenosine (m6A) in mammalian mRNAs, and simultaneous detection of N1-methyladenosine (m1A) and m6A in human mRNAs.